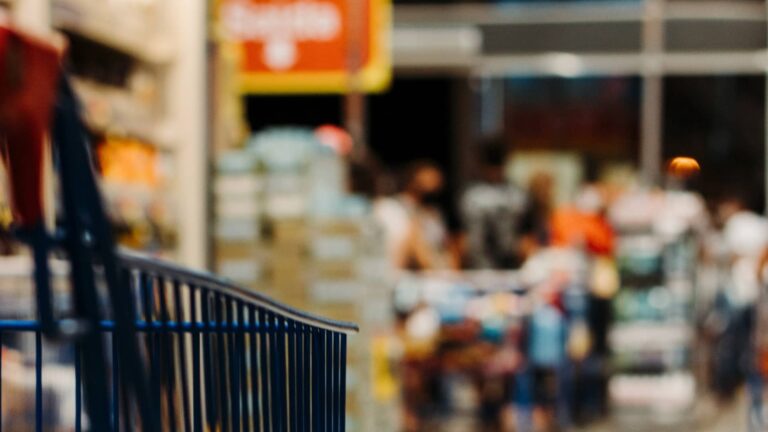
Around the time ARkit came out in 2017, the tech press fawned over a video that showed an AR wayfinding app in a grocery store. Through the phone’s viewfinder, one could navigate to items on their grocery list, opening everyone’s eyes to AR’s mix of magic and practicality.
But there was one issue: the app didn’t exist. Like a concept car, it was a design with a flashy front-end UX, but no back-end functionality that was available anywhere in a real product. Building that grocery-store wayfinding app has since remained a vexing and unsolved problem.
The biggest issue is data. Without going too deep into the history, players such as Krillion, NearbyNow, Milo and even Google Shopping have assembled point-of-sale (POS) data to come fairly close to bringing this vision to life, albeit in more of a 2D search-engine context.
Beyond challenges in tapping into the right data is the reality of a quickly changing world. In other words, real-time inventory is constantly changing, especially in a category like grocery stores with thousands of low-margin products that encounter high levels of daily turnover.
Collectively & Passively
Against that backdrop, a serious contender recently emerged to bring this grocery-wayfinding vision to life. Founded by former Niantic exec Ross Finman (more on that in a bit), Augmodo is using computer vision to map store shelves as a data source for in-store navigation.
To pull this off, the company plans to work directly with retailers to equip store personnel with body cams that scan and assess store shelves as they go about their business. The thought is that they can collectively and passively capture holistic 3D scans of a given store and its shelves.
The result is what the company calls a realogram, which becomes the basis of a few outputs. Chief among them could be a consumer-facing wayfinding app. Realogram data could also be fed into an API (our speculation) or otherwise provided to retail apps or players like Google Maps.
Beyond these consumer-facing endpoints, Augmodo’s realograms could be useful in a B2B sense. We’re talking data to optimize retailers’ ordering, logistics, or just-in-time inventory systems. There’s also the art of merchandizing, which is the science of where to place products.
Origin Story
Back to Finman, Augmodo was inspired by a personal experience when he and his wife spent hours driving hundreds of miles to find baby formula during the product’s well-known 2022 shortage. Held down by a lack of inventory transparency, he knew there had to be a better way.
Beyond Augmodo’s inception, other missing pieces came from his background. At Niantic, he helped drive the company’s “planet-scale” mapping endeavors. In short, it builds 3D maps of popular waypoints by crowdsourcing requisite scanning to legions of Pokémon Go players.
Those 3D maps are critical for the real-world interactivity of its games – not just Pokémon Go but its Lightship platform for game developers. Like autonomous vehicles need LiDAR scans to “see” the road, immersive geospatial experiences need to know the geometry of their surroundings.
Augmodo is also analogous to Google Live View. which uses the smartphone camera to localize a given device. Cross-referencing that camera feed with its Street View image database, Google can know where you’re standing and then overlay 3D directional arrows to your destination.
Stress Test
The common thread in all the above is using the camera as a sensor. Applied to the challenge of retail POS data, it’s a novel approach. Challenges could await in forming retailer partnerships, but Augmodo could also scale its efforts by crowdsourcing to shoppers, a la Niantic or Waze.
Meanwhile, the self-funded company is off to a good start with six people joining Finman from Standard AI, Microsoft, Apple, and Tesla. And it’s already landed its first pilot – a small store in New York with deals in the works with a 2,000-store chain and another 500-store chain.
In the longer term, the underlying technology seems expandable to other verticals such as apparel. Until then, grocery is a logical starting point given the size of its addressable market, and the fact that its inventory complexity makes it the best place to stress-test the platform.
“We’re targeting grocery to begin with,” Finman told GeekWire, “because they have the highest throughput and largest workforce, so they have the biggest real-world data problems […] You may only order a sweater from Macy’s twice a year. You order groceries twice a week.”
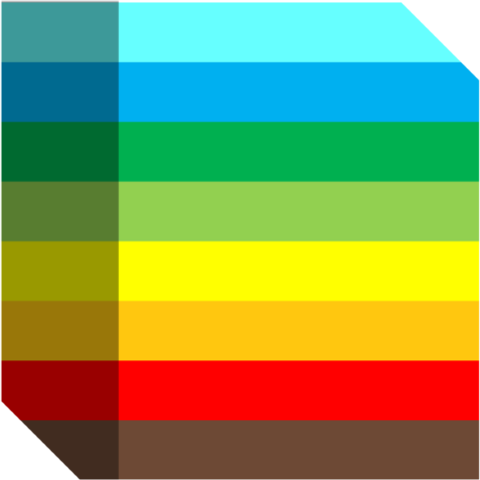